Machine Learning as a Service (MLaaS): A Complete Guide 2024. With machine learning as a service (MLaaS), customers may tap into the capabilities of machine learning through user-friendly, scalable, and affordable cloud-based solutions, making advanced analytics more accessible than ever. With MLaaS, businesses can access advanced data processing and predictive analytics without complex installations or specialist knowledge. It eliminates the conventional obstacles to entrance into complex data analysis and is both scalable and inexpensive.
Adding blockchain technology and MLaaS makes it even more appealing and trustworthy, crucial for protecting sensitive data. Thanks to blockchain’s capacity to guarantee data integrity and traceability, the MLaaS platform becomes an even more dependable option for consumers. This combination of technologies has the potential to simplify processes and lay a solid groundwork for machine learning projects, allowing for better, more deliberate decision-making in a data-driven society.
Understanding Machine Learning as a Service
As a subset of cloud computing, MLaaS encompasses a variety of services that provide access to machine learning resources. Machine learning as a service relies on data preprocessing, automated data transformations, model training, model evaluation, and prediction. These components allow users to build, release, and manage machine learning models without spending money on the specialized hardware generally needed for these operations.
In MLaaS, data preparation refers to the steps used to prepare data for usage in machine learning models, such as cleaning and formatting. During model training, the service feeds data into an algorithm to learn to spot trends and make judgments. After training, it goes through a review to make sure the model is correct and valuable. Predictions based on fresh data can be made once a model is considered satisfactory. Last, automatic data transformations ensure the trained models get data in a format they can understand and process well.
Augmenting MLaaS’ Effectiveness through Blockchain
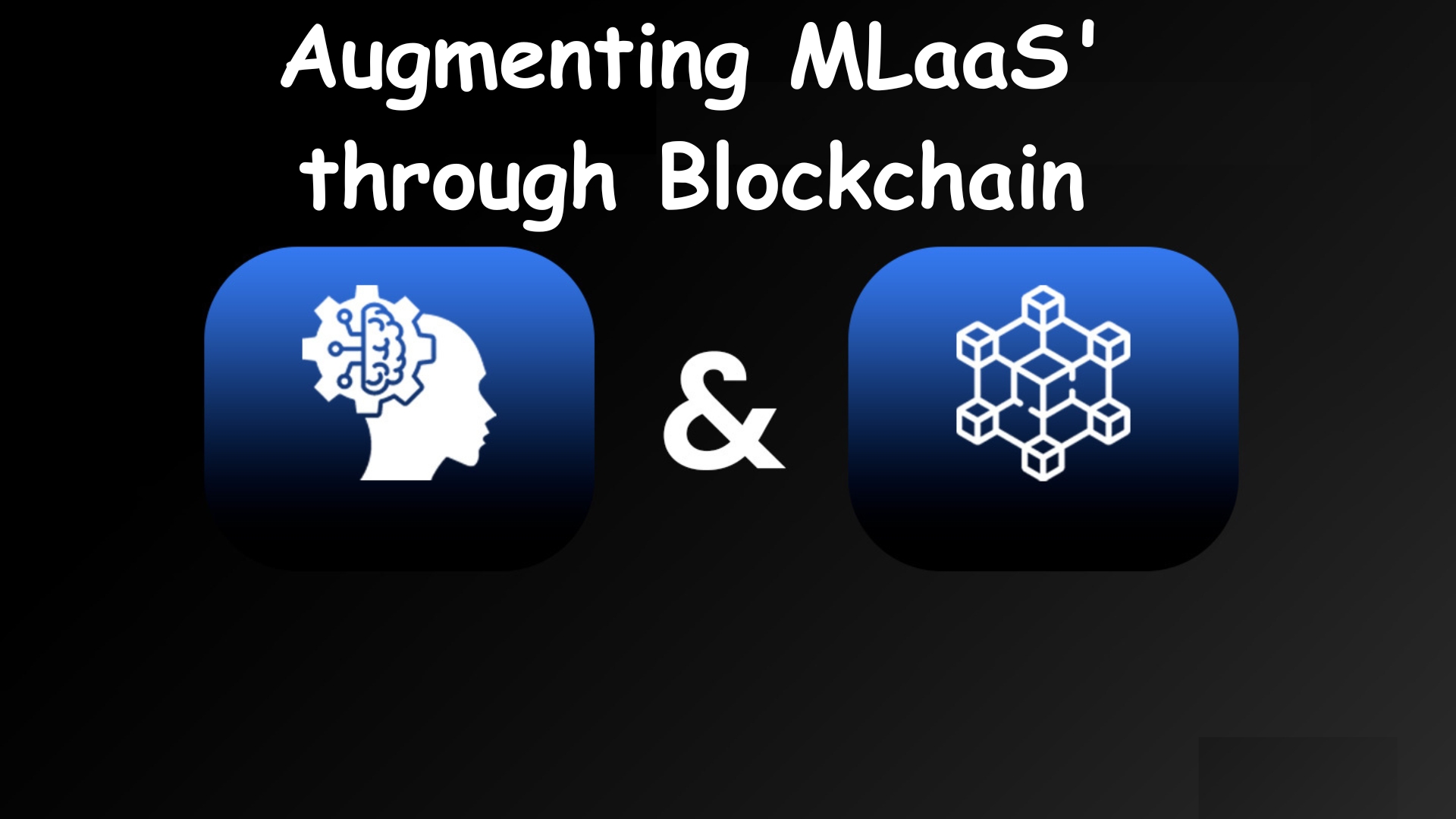
With blockchain technology’s transparent and secure data exchange environment, MLaaS can be much more effective. The blockchain’s distributed ledger architecture guarantees the immutability and traceability of data shared across networks. This is crucial for preserving the integrity of data sets, particularly training data, utilized in machine learning.
They allow many parties to share data securely and transparently while protecting users’ privacy. Smart contracts built on the blockchain can automate the terms of model access and data usage, guaranteeing compliance and equitable pay. Collaborative learning without revealing private information is also possible with solutions like blockchain-based decentralized model training and federated learning.
Essential for regulatory compliance, blockchain’s ledger architecture simplifies tracking data’s provenance and usage history. But to implement it successfully, we need to solve the problems of scalability, interoperability, and integration. However, there is significant potential for data-driven operations to transform through the integration of blockchain and MLaaS, all while upholding standards of transparency and security.
Rise of Cloud-based MLaaS
Data science has reached a tipping point with the arrival of cloud-based MLaaS. Providers such as Amazon Web Services’ SageMaker, Google Cloud AI, and Microsoft Azure Machine Learning have democratized access to advanced data science tools by offering machine learning capabilities as a cloud service. Data storage, preprocessing, model development, training, and deployment are all part of the end-to-end machine-learning ecosystem provided by these platforms. Scalability, flexibility, and lower administrative expenses are some of the advantages of this strategy.
For example, AutoML is a service offered by Google Cloud AI that lets users train high-quality models specific to their business needs, even if they don’t have much experience with machine learning. Data scientists can also use AWS SageMaker, a fully managed service, to rapidly construct, train, and deploy ML models. These services can quickly adapt to a growing workload by modifying their cloud resources, ensuring optimal performance.
Seamless ML model deployment using MLaaS
With the introduction of MLaaS, a crucial step in the data science pipeline—the deployment of machine learning models—has become much more manageable. The MLaaS platform makes it easier and faster for companies of any size to go from developing a model to putting it into production. It can be time-consuming and expensive to build up servers, manage dependencies, and ensure scalability for traditional model deployment. But MLaaS systems hide all those complexities so businesses can deploy their models with a few clicks.
Automated model tuning, one-click deployment, and easy monitoring of deployed models are just a few of the ways in which services like AWS SageMaker, Azure ML, and Google AI Platform have transformed this process. By connecting directly to Amazon Elastic Compute Cloud (EC2) instances, SageMaker customers can rapidly deploy their trained models and adjust the compute resources needed based on changes in traffic demands. Thanks to this elasticity, companies can keep their models running well without breaking the bank on new hardware.
MLaaS for Predictive Analytics
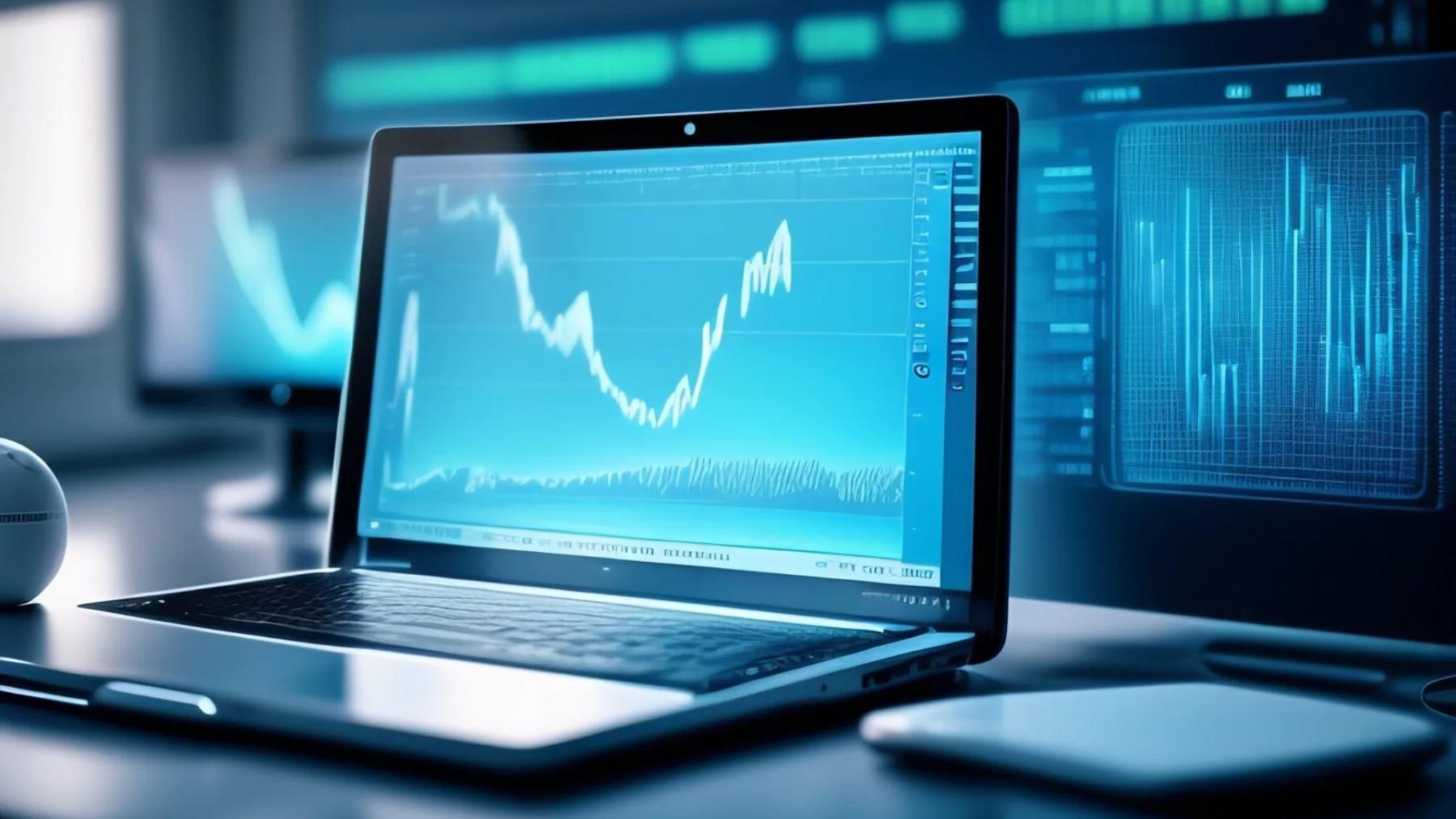
For companies looking to include predictive analytics in their long-term strategies, MLaaS has become an indispensable tool. Companies can gain a competitive advantage and improve decision-making with the help of MLaaS, which allows them to foresee outcomes using past data. To avoid expensive downtimes, a logistics company may use MLaaS to forecast when a fleet repair is required, or a streaming service could use it to anticipate user preferences for personalized content recommendations.
The complicated task of creating predictive models is made easier using MLaaS systems. To make predictive analytics more accessible, they automate essential procedures like feature selection, model training, and validation. Businesses can now access insights previously only available to larger enterprises with data science teams, even without extensive analytics knowledge.
Real-world Examples of MLaaS
Machine learning as a service (MLaaS) has become an industry-wide game-changer, providing a flexible platform for companies to harness AI’s potential. Machine learning as a service (MLaaS) technologies, such as Amazon’s Forecast service, optimize supply chains, improve consumer experiences through personalized product suggestions, and predict product demand in the retail industry. Efficiency and consumer happiness can both see huge boosts with this predictive capability.
Industries that cannot compromise on data integrity are witnessing remarkable progress in blockchain-enhanced MLaaS. By combining MLaaS with blockchain, the agriculture business can monitor the supply chain from farm to table, ensure transparency, and manage quality. This allows them to analyze crop yields, predict optimal planting periods, and more. The IBM Food Trust uses this technology to guarantee the safety of food delivery routes.
Machine learning as a service (MLaaS) is changing the game for financial institutions when managing risk and detecting fraud. When proactively detecting and avoiding fraudulent actions, Mastercard employs MLaaS to analyze real-time transaction data, giving them a predictive edge. Similarly, algorithmic trading—which uses predictive models to analyze market data and trade automatically—is another usage of MLaaS by financial institutions and investment firms.
Pros and Cons of MLaaS
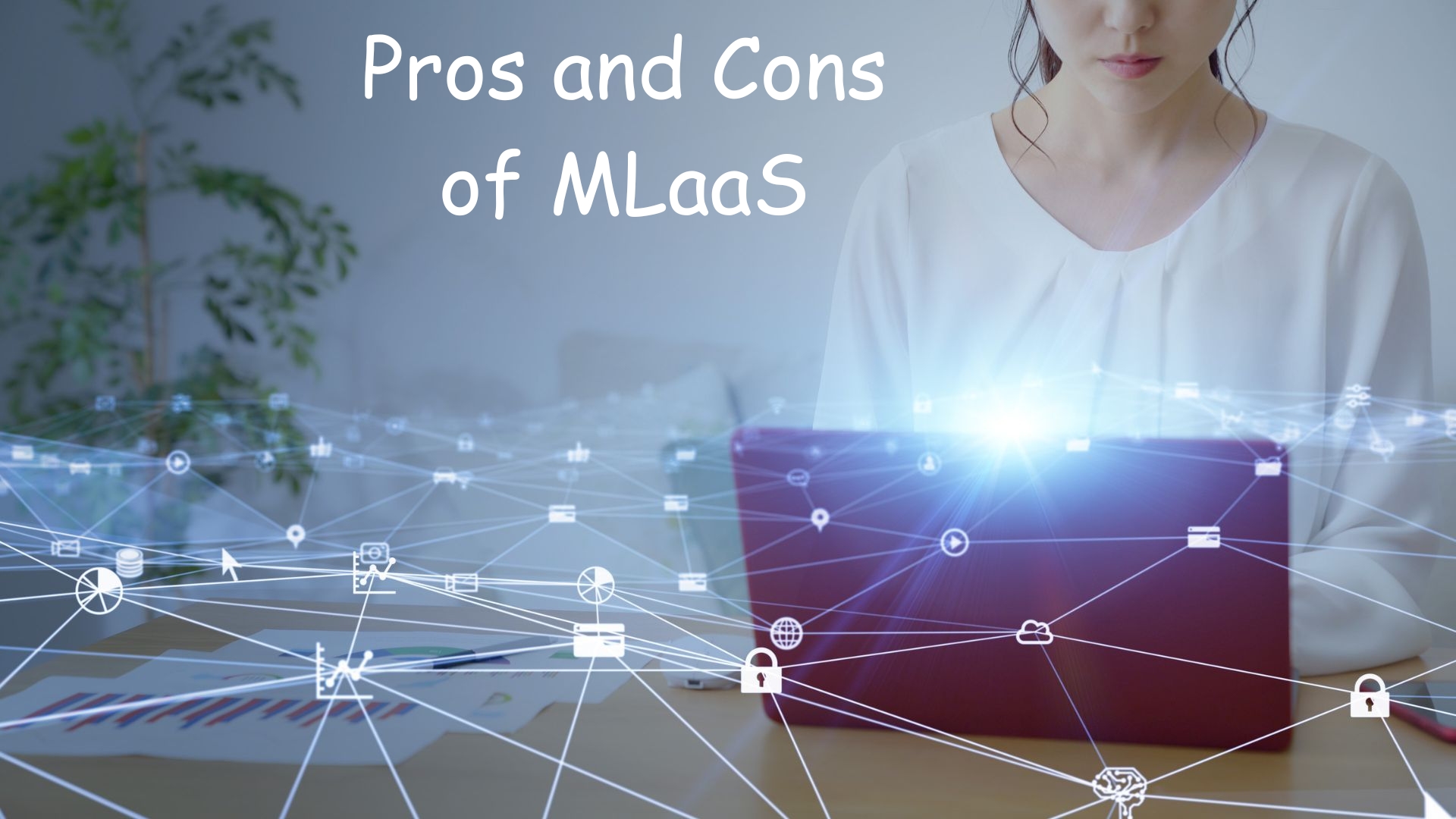
MLaaS provides access to machine learning through a cloud service platform, doing away with the requirement for costly hardware and trained employees. As a result, companies of all sizes will have equal access to cutting-edge analytical tools. Businesses can save money with this strategy since they pay for the services they utilize, usually through subscriptions. This method eliminates huge initial expenditures while still letting you effectively manage your budget. Businesses, particularly those experiencing rapid expansion or fluctuating demand, can benefit significantly from the adaptability of the pay-as-you-go approach, which allows for seamless scalability to meet their specific requirements.
But MLaaS isn’t without its flaws. The storage and processing of sensitive information on external servers raises concerns about data security. Another potential danger is relying too much on the service provider. In addition, MLaaS solutions might not provide the same degree of personalized service as a custom-made, in-house solution, which could lead to restrictions in terms of control and customization.
Future of MLaaS
A growing trend toward ubiquitous use across many sectors is on the horizon for MLaaS. From healthcare diagnostics to improved customer service personalization in retail, MLaaS is poised to revolutionize a diversity of industries that have hesitated to adopt the technology due to its high cost and increasing accessibility.
We may anticipate that future innovations will greatly enhance automation and personalization capabilities, resulting in interactions and decision-making that are far more complex. Developments will improve virtual communication in natural language processing, and real-time analytics will be accelerated by integrating MLaaS with emerging technologies like the Internet of Things (IoT) and edge computing.