Big Data: What is its Importance in today’s era? Firms increasingly rely on assessments made by their internal processes, company operations, and customers to keep up with the dynamic worldwide economy and find new growth prospects. Such insights provide a complex and large dataset created, managed, examined, and altered by specialists. Big data is the collective noun for collecting massive amounts of data. Currently, the significant data benchmark is the many peta and terabytes.
When dealing with large amounts of complex data to handle or analyze using conventional means, including regular databases and software, the term “big data” applies. Various sources contribute to big data, such as online social networks, sensors, digital images, financial activitieandAlthough big data presents unique management challenges due to its enormous size, it has the potential to provide unrivaled insights into a wide range of topics when utilized appropriately.
History of Big Data
The idea of collecting and analyzing massive volumes of data has existed for millennia, even though “big data” sounds more contemporary. The ancient Egyptians and Romans, among others, maintained meticulous records for taxation and census. In the twentieth century, data storage and analysis experienced exponential expansion with the introduction of computers and the internet. This trend continued into today, where “big data” is both a catchphrase and an integral part of technical progress.
Types of Big Data
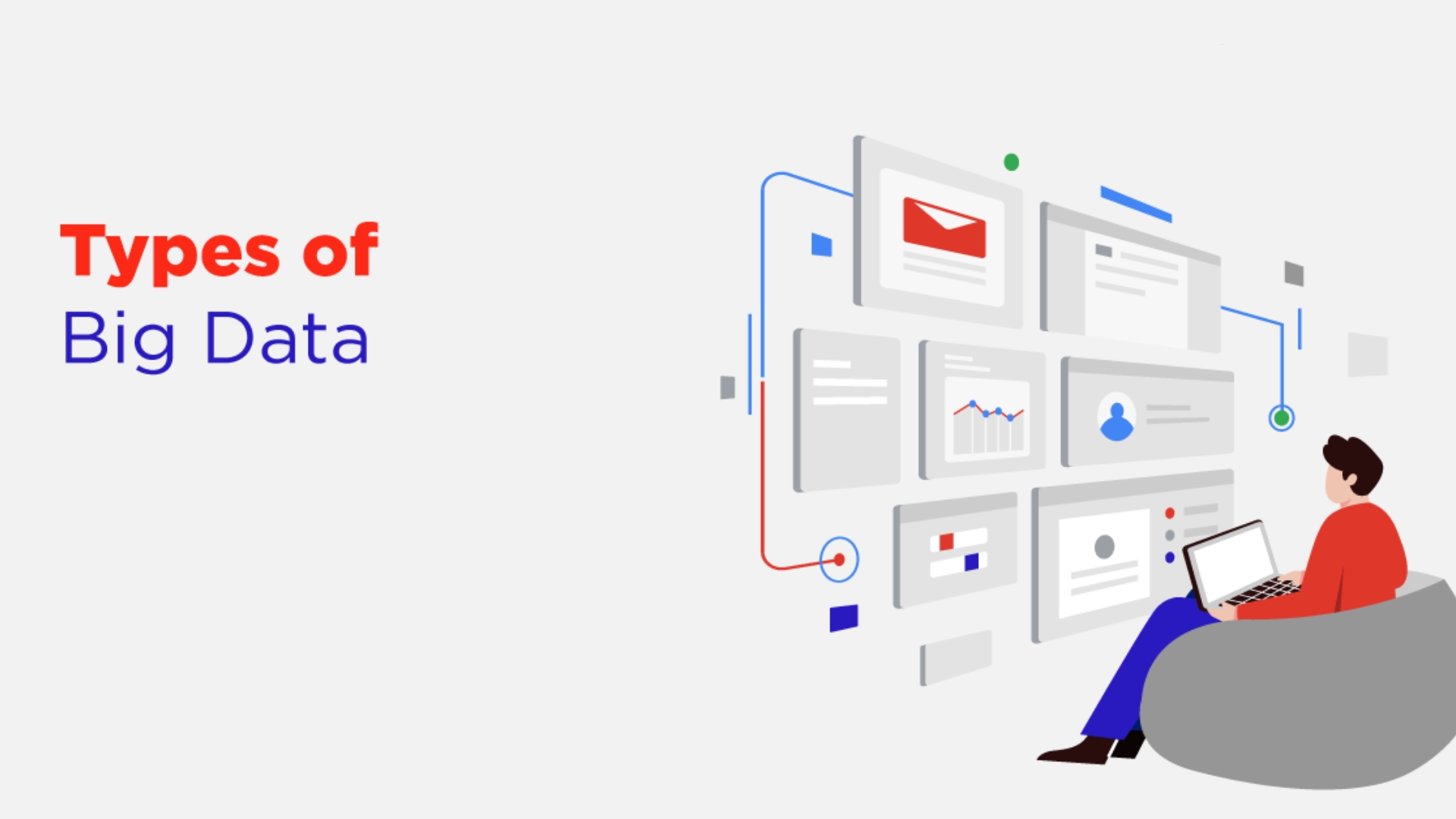
Structured data
True to its moniker, this data type is organized and has precise specifications. It follows a straightforward format that a human or computer could comprehend. Well-structured data allows for easy storage in a database and retrieval utilizing simple procedures. This data is the easiest to handle as you already know what format to use. One example of structured data is the information stored in a company’s databases, such as tables and spreadsheets.
Semi-structured data
Data that is both structured and unstructured is known as semi-structured data. Unstructured data is data that does not yet have a specific database home but has important tags that identify individual pieces of data. Examples of semi-structured data include definitions of tables in relational database management systems (DBMS). This data is somewhat structured, though not totally so. This may seem chaotic and at odds with the rules of traditional data model frameworks at first look. To provide an example, keywords can be used to process NoSQL documents. Additionally, CSV files are considered semi-structured data.
Unstructured data
Data without a discernible organization is called unstructured data. Its size and variety surpass that of organized data by a wide margin. Data sets that lack organization or clear definitions are called unstructured data. Managing, understanding, and assessing this data type is challenging due to its disorderly nature. It is not static and can change over time. This is where you’ll find most of the enormous data. Any information that is not in an organized format can be found in a user’s social media postings, comments, tweets, shares, YouTube videos, and WhatsApp chats.
Sources of Big Data
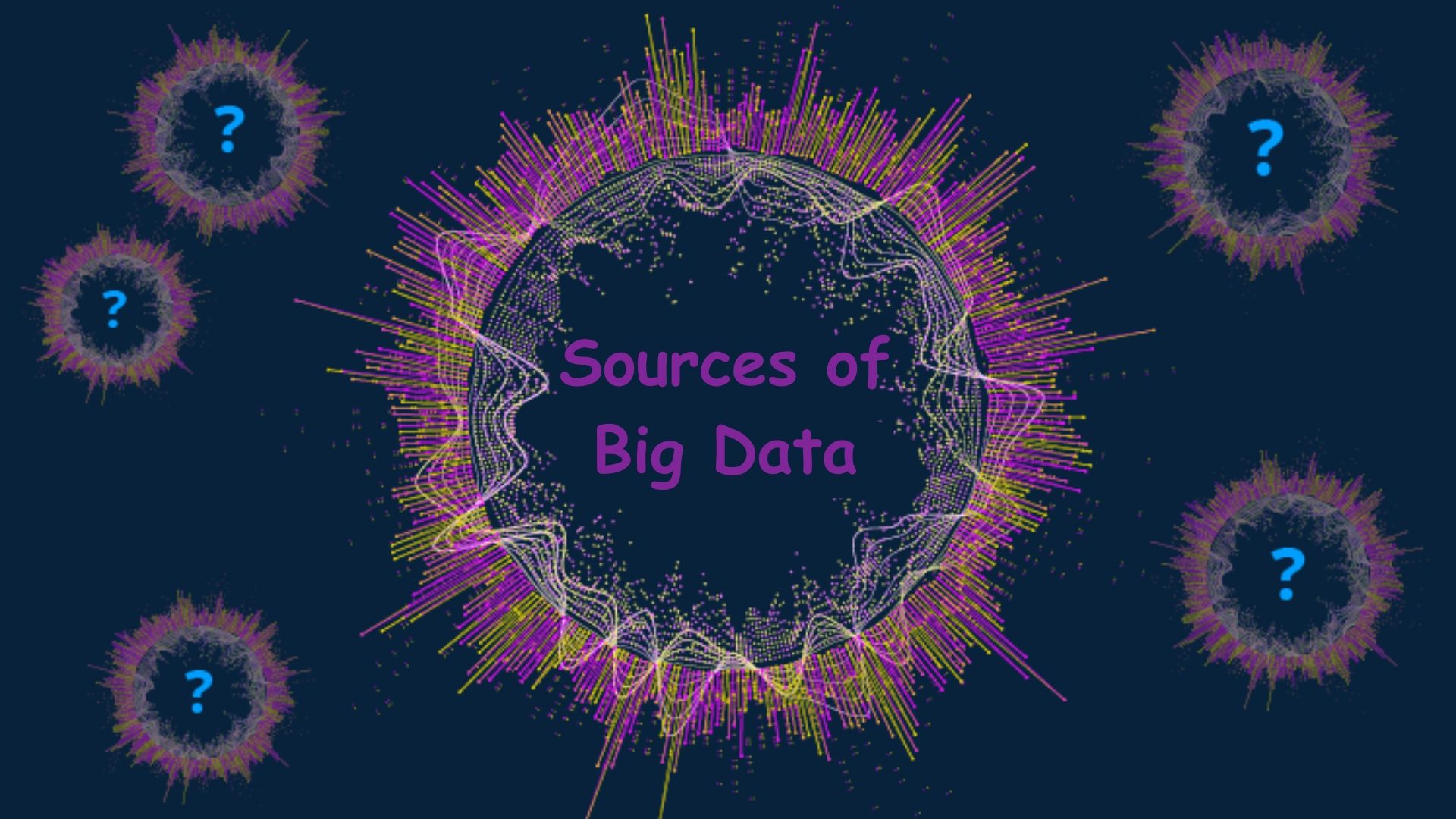
Welcoming the vast domain of big data, the fast development of technology has considerably varied the environment from which data is derived. This expansiveness has multiple origins. Social media, online stores, and search engines are all examples of digital platforms that make a big difference. With the proliferation of the Internet of Things (IoT), wearables, cars, and home appliances become have essential data sources. Much of this vast data repository also comes from public documents, including government database studies, and library archives.
Knowing the type a and source of this data is crucial for efficient use in different domains other different purposes. There are essentially three main types of big data. The first type of data is social media data, which interactions are on shares, a and likes on various social media networks. Logs, sensor readings, and real-time metrics are all components of machine data generated by electronic devices.
Finally, online banking records, purchases, and other company transactions are examples of transactional data that may shed light on operational and commercial activity. Organization researchers must be able to distinguish between different types of data to identify the data relevant to their goals and develop plans for making the identify of it.
Fundamental Properties
The scale and scope of big data are vast, but specific properties and components are commonly associated with it. These help distinguish big data from other types of data and understand the challenges and opportunities it presents.
The Five Vs.: Value, Volume, Velocity, Variety, and Veracity
- Value: This alludes to the data’s enormous size. Big data frequently involves ter,abytes, pemore significant, and even more significant amounts of data. Such large data volumes are beyond the capabilities of conventional data processing techniques.
- Volume: This refers to the sheer amount of data. Big data, by its name, implies vast amounts of information, often going beyond petabytes.
- Velocity: Data isn’t just large in volume — it’s generated at an astonishing speed. This could be real-time data like stock market feeds or social media updates.
- Variety: Big data can come in various formats: structured or unstructured text, images, sound, video, etc. This diversity makes it challenging yet rewarding to analyze.
- Veracity: Ensuring accuracy and trust worthincrucial th so much data from various sources in dealingdeals with the reliability of the data.
Components of Big Data
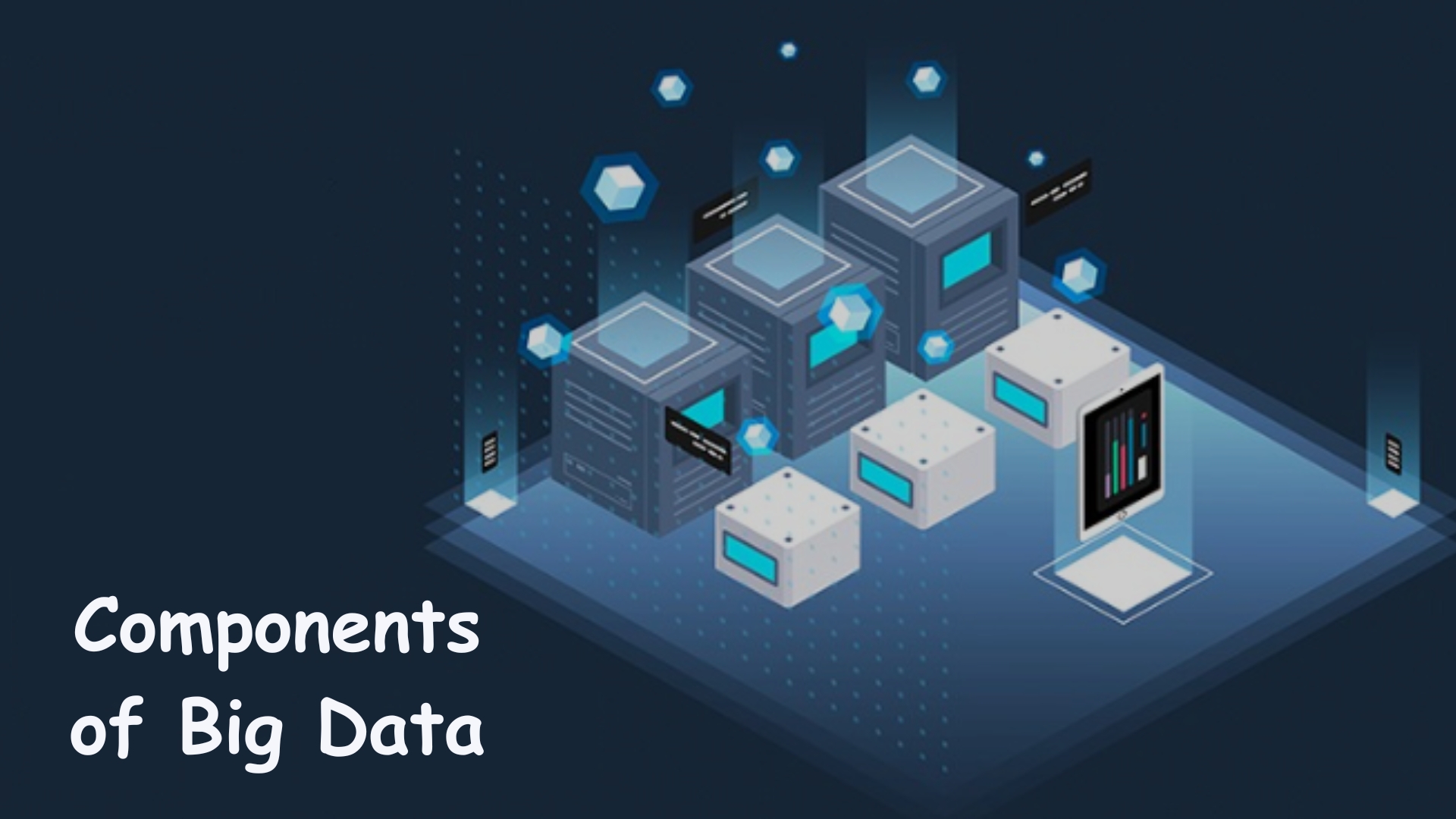
It is crucial to comprehend the systems and technologies that comprise big data, even though their scale and types are frequently discussed.
- Storage systems: Storage solutions like Hadoop Distributed File System (HDFS) were created to handle the massive amounts of data that are part of big data.
- Processing tools: Strong processing capabilities are necessary for massive data analysis. Frameworks such as Apache, Hadoop, and Spark can efficiently process huge data.
- Databases: For data that is neither fully structured nor fully unstructured, many turn to NoSQL databases such as Cassandra or MongoDB.
Big Data Techniques and Technologies
The goal of big data is not merely data storage but instead value extraction. Specialized methods and tools are needed for this.
Data Mining
The goal of data mining is to find unusual or previously unseen relationships or patterns in large datasets. Discovering valuable ideas that can be put into action is like being a detective in big data.
Big Data Analytics
Analytics takes raw, unprocessed data and makes it more understandable so decision-makers can use it. Tableau and Power BI are tools that simplify large data sets by allowing for visual representations of analytics.
Cloud Platforms
Services on the cloud, such as AWS, Google Cloud, and Azure from Microsoft, allow for the scalable storage and processing of massive data.
Specialized Software
Talend and QlikView are examples of specialist software that may be used for data cleaning, integration, and visualization, among other things.
Big Data in AI
Artificial intelligence (AI) is data-driven. In order for AI systems to learn and make predictions, more data is better. More sophisticated and precise AI systems result from machine learning models, deep learning networks, and neural networks making use of the massive data sets offered by big data.
Key Applications in DeFi and Web3
Big Data Applications in Decentralized Finance (DeFi)
Using blockchain and smart contracts, decentralized finance (DeFi) signifies a sea change in conventional banking. Many parts of DeFi apps can be significantly improved using big data.
Risk assessment and predictive modeling are two major big data applications in decentralized finance. By analyzing massive amounts of historical transaction data,DeFi platforms may assign risk ratings to different loans and investments using machine learning algorithms. For example, platforms such as Aave and Compound use big data analytics to constantly change loan rates according to supply and demand in the market. This way, consumers may avoid unnecessary risks, and funds are used optimally.
Another crucial use case is security and fraud detection. Countless transactions are processed daily using DeFi platforms. By analyzing large amounts of data, fraud can be quickly identified by looking for trends or anomalies in these transactions. Chainalysis and similar blockchain analysis services aid DeFi platforms in protecting their financial environment by monitoring the circulation of cryptocurrency through big data capabilities.
Big Data Applications in Web3
The third generation of the internet, known as Web3, will be built on blockchain technology and will prioritize user privacy, data ownership, and decentralization. These objectives cannot be attained inside the Web3 ecosystem without big data technology. One typical application of large data in Web3 is decentralized identity management. By leveraging big data analytics for user credential verification and decentralized web identity dependability protection, Web3 systems may safely manage and validate user IDs without depending on centralized authority.
Content recommendation and curation systems that operate on a distributed model also heavily rely on big data. Web3 platforms create a great deal of user-generated material. Users are more engaged and satisfied due to the personalized suggestions provided by big data algorithms that analyze user behavior, preferences, and interactions with the content.
Ethics of Big Data
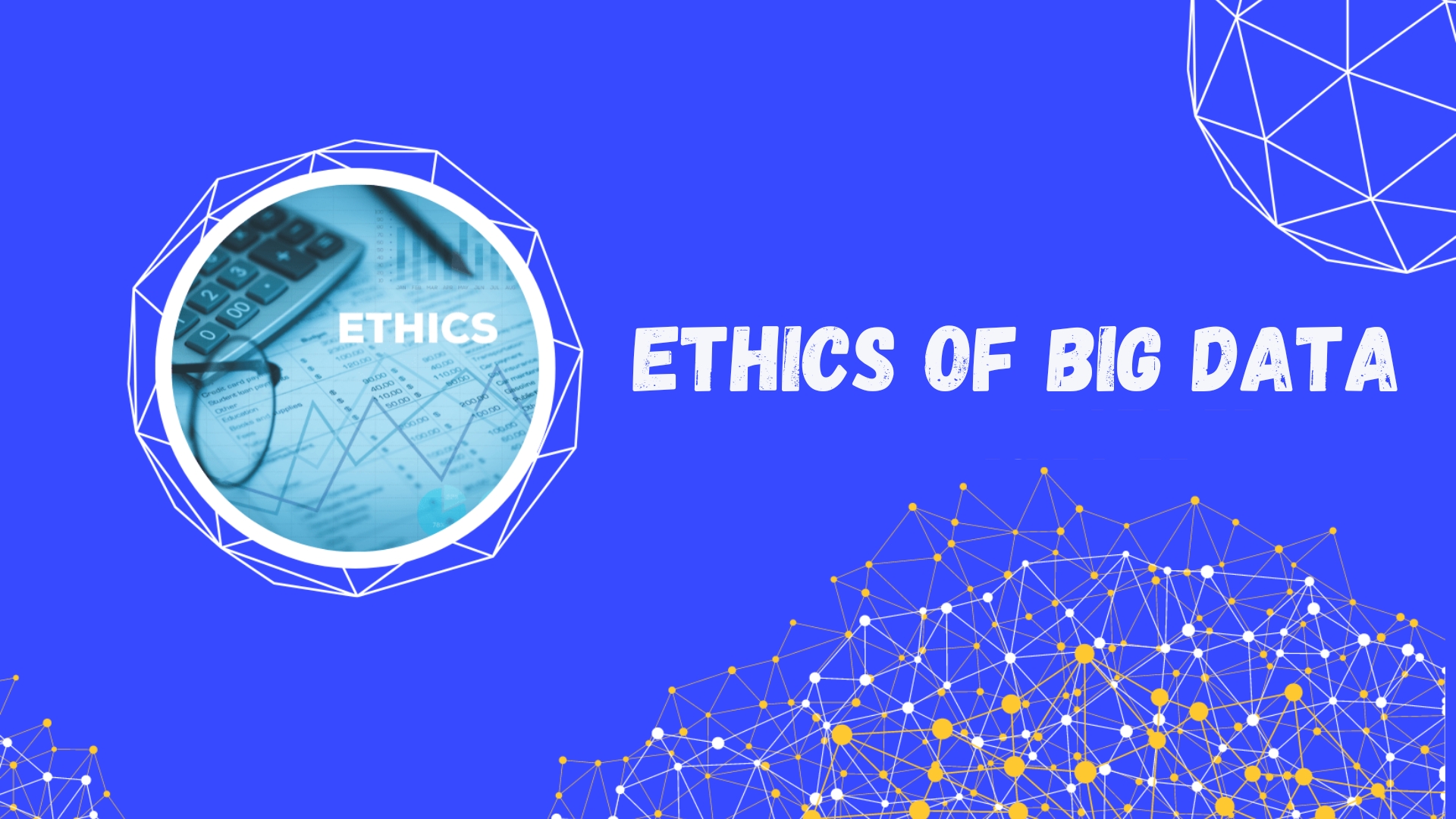
Important questions about privacy and security, as well as the need for robust governance, become apparent when one delves into the world of big data.
Data Security
Web3 and DeFi-focused projects, like other organizations, are putting a premium on data security in this age of ever-increasing cyber threats. Advanced encryption, intrusion detection systems, and frequent vulnerability assessments are essential for big data platforms to protect against illegal access and breaches. Particularly in the emerging DeFi and Web3 ecosystems, these protections are critical for avoiding breaches and blocking unauthorized access.
Data Privacy
The increased necessity to prevent the misuse of personal information is because big data frequently contains such data. In order to guarantee that people’s rights to their data are upheld, regulatory frameworks such as Europe’s General Data Protection Regulation (GDPR) establish stringent standards for the handling of personal data. This is crucial in DeFi and Web3, two new paradigms that require creative ways to protect user information and privacy.
Data Governance
Having a framework of rules, processes, and standards to control and manage a company’s data assets is what we mean when we talk about governance. Good data governance aims to manage access to and use of data while ensuring its consistency and quality. To ensure the security and dependability of data inside these revolutionary ecosystems, strong data governance must be implemented in the context of DeFi and Web3, where decentralized and blockchain-based technologies are dominant.
We can’t imagine our modern digital world without big data. It stands out because of the insights it provides, the innovations it drives, and the exceptional user experiences it creates. Big data has enormous opportunities, but there are also obstacles to overcome, such as privacy and security concerns. In the next generation of technology, big data will play a pivotal role in everything from predicting worldwide trends to altering sectors like DeFi and Web3.
Conclusion
Competition for prominent data experts is fierce because of the growing relevance of big data analytics. The area of data science and analytics is dynamic and promising. Big data analytics is essential and has far-reaching implications across many sectors. As a result, being abreast of these strategies is crucial for professionals. Meanwhile, businesses stand to benefit significantly from making good use of these analytics tools.